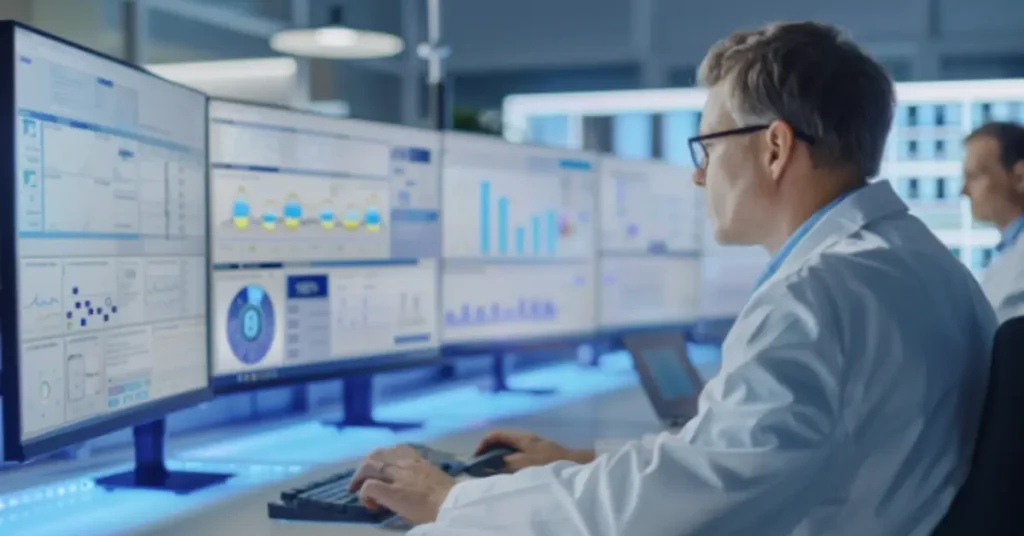
In this article:
- What Is Data Management in Clinical Research?
- Key Objectives of Clinical Data Management
- The Clinical Data Management Process: Step-by-Step
- Common Challenges in Clinical Data Management and Effective Solutions
- Best Practices for Effective Clinical Data Management
- 1. Implement Robust EDC Systems for Accurate and Real-Time Data Collection
- 2. Conduct Regular Data Audits to Maintain Quality and Integrity
- 3. Utilize Automated Data Validation Tools to Minimize Errors
- 4. Train Research Teams on Data Management Protocols and Regulatory Requirements
- 5. Ensure Compliance with Data Privacy Laws and Adopt Cybersecurity Measures
- Emerging Trends in Clinical Research Data Management
- The Importance of Robust Data Management in Clinical Research
Data management in clinical research is the backbone of every successful clinical trial. Without proper data collection, validation, and security, research findings become unreliable, delaying life-saving treatments and leading to regulatory setbacks. Accurate data is essential for patient safety and medical progress.
In this article, we’ll explore what clinical data management is, why it matters, and how the process works. You’ll learn about key steps, from data collection to regulatory submission, along with the biggest challenges researchers face. We’ll also highlight best practices and emerging technologies that enhance data accuracy and security.
However, clinical trials generate vast amounts of data, and even minor errors can result in costly delays or compliance issues. Researchers, sponsors, and data managers must navigate challenges related to accuracy, security risks, and evolving regulations.
What Is Data Management in Clinical Research?
Data management in clinical research is the process of collecting, storing, verifying, and protecting clinical trial data to ensure accuracy, reliability, and compliance with regulations. It plays a critical role in transforming raw data into meaningful results that regulatory authorities and healthcare professionals can trust.
Every clinical trial generates vast amounts of information—from patient records and laboratory results to adverse event reports. Without a structured system to manage this data, errors, inconsistencies, and security risks can compromise the integrity of the study.
Clinical data management (CDM) ensures that all data is accurate, complete, and securely maintained, allowing researchers to make informed decisions and regulatory agencies to approve new treatments with confidence.
Key Objectives of Clinical Data Management
The primary goal of clinical data management is to produce high-quality, error-free data that meets regulatory standards. To achieve this, CDM focuses on several key objectives:
- Data Accuracy – Ensuring that all data points entered are correct, consistent, and verifiable to prevent errors that could impact study results.
- Data Completeness – Making sure no critical information is missing so the study remains valid and meets compliance requirements.
- Data Integrity – Maintaining the reliability of data throughout the trial, preventing unauthorized modifications or inconsistencies.
- Data Security – Protecting sensitive patient information from breaches or unauthorized access by following strict security protocols.
- Regulatory Compliance – Adhering to guidelines set by organizations like the FDA, EMA, HIPAA, and GDPR to ensure ethical and legal data handling.
By focusing on these objectives, clinical data management helps safeguard the credibility of research findings, supports faster regulatory approvals, and ultimately leads to safer, more effective medical treatments for patients.
The Clinical Data Management Process: Step-by-Step
Clinical data management (CDM) follows a structured process to ensure that every piece of data collected in a clinical trial is accurate, complete, and regulatory-compliant. From the moment data is recorded to the final submission to regulatory agencies, each step is crucial for maintaining the integrity of a study.
Below, we break down the key phases of clinical data management.
1. Data Collection in Clinical Research
Clinical trials generate data through various methods, including:
- Electronic Data Capture (EDC) Systems: These digital platforms allow researchers to collect and facilitate real-time data, reducing errors and improving efficiency.
- Paper-Based Data Collection: While less common today, some studies still rely on traditional paper forms, which require manual entry into digital systems later.
- Wearable Devices and Remote Monitoring: Smartwatches, mobile apps, and other digital tools collect patient data continuously, providing real-time insights.
- Decentralized Clinical Trials (DCT) Technologies: Data collection happens remotely via Mobile apps, electronic patient-reported outcomes (ePRO), eDiary tools, and Telemedicine platforms.
- Electronic Health records (EHRs): Pulls real-world data directly from clinical care systems.
- Patient-Report Outcome Measures (PROMs): Collected via digital or paper questionnaires. Measures how patients feel or function in relation to their health condition.
The industry is shifting toward digital data collection technologies due to their ability to minimize human error, enhance data security, and streamline trial workflows. These tools also support regulatory compliance by maintaining detailed audit trails of all data entries.
2. Data Entry and Validation
Once collected, data must be entered into a database and validated to ensure its accuracy. This step is essential because even minor errors can impact study results.
Common data validation techniques include:
- Double Data Entry: Two individuals enter the same data separately, and discrepancies are flagged for review.
- Automated Validation Checks: EDC systems detect missing fields, duplicate entries, and logical inconsistencies.
- Manual Review: Data managers cross-check records against source documents to confirm accuracy.
Effective data validation helps maintain reliability and regulatory compliance, reducing the risk of errors that could delay trial approval.
3. Data Cleaning and Reconciliation
Even with strong validation measures, discrepancies can arise with the data collected during clinical trials. Data cleaning ensures that all collected information is consistent, accurate, and complete before analysis.
Key data cleaning steps include:
- Identifying and correcting inconsistent or missing data.
- Resolving discrepancies between data sources (e.g., comparing patient-reported outcomes with lab results).
- Standardizing data formats for uniformity.
Data reconciliation ensures that all versions of the same data align across different systems and databases. This step is critical for maintaining a single, trusted source of truth in clinical research.
4. Data Storage and Security Measures
Clinical data contains sensitive patient information, making security a top priority. Secure storage practices prevent data loss, breaches, or unauthorized access.
Best practices for data security include:
- Encrypting data during storage and transmission
- Using role-based access controls to limit who can view or edit data
- Always backing up to avoid data loss caused by any system failure
Compliance with HIPAA (Health Insurance Portability and Accountability Act), GDPR (General Data Protection Regulation), and other data privacy laws ensures that patient information is protected throughout the trial.
5. Data Submission and Regulatory Compliance
The final step in the clinical data management process is submitting trial data to regulatory authorities like the FDA (Food and Drug Administration) and EMA (European Medicines Agency) for review.
To meet Good Clinical Practice (GCP) guidelines, data submissions must be:
- Accurate: Free from errors or inconsistencies.
- Complete: Containing all necessary clinical and safety information.
- Audit-Ready: Traceable and documented to demonstrate compliance.
Successful data submission is essential for securing regulatory approval and ensuring that new treatments can move forward in the approval process.
Common Challenges in Clinical Data Management and Effective Solutions
Managing clinical trial data is a complex task that requires efficient data collection, validation, storage, and analysis. Moreover, there are metrics in clinical data management (e.g., data access logs for security and query rate for data quality) that data managers must be aware of to improve data accuracy, streamline workflows, and ensure regulatory compliance.
Several challenges can impact the data management process, leading to delays, errors, and compliance risks. Below are the most common challenges in clinical data management and practical solutions to overcome them.
Data Integrity Issues
Challenge:
Ensuring data accuracy, completeness, and consistency is critical in clinical research. Missing data, duplicate entries, and human errors can compromise data quality, affecting study outcomes and regulatory approval.
Solutions:
- Use electronic data capture (EDC) systems to minimize manual entry errors and improve data accuracy.
- Implement data validation rules to flag incorrect or incomplete data during data capture.
- Conduct real-time data monitoring to detect inconsistencies early.
- Establish a comprehensive data management plan to outline data management activities and ensure data integrity throughout the clinical trial process.
Regulatory Compliance Complexities
Challenge:
Regulatory guidelines set by agencies like the FDA, EMA, and the Society for Clinical Data Management (SCDM) constantly evolve. Good Clinical Practice (GCP) and standards such as the Clinical Data Interchange Standards Consortium (CDISC) and Clinical Data Acquisition Standards Harmonization (CDASH) must be followed to ensure compliance.
Failing to meet these standards can result in trial delays or rejection.
Solutions:
- Train clinical research associates, data managers, and study teams on the latest regulatory updates.
- Use a clinical data management system (CDMS) that adheres to CDISC and GCP standards.
- Maintain audit trails to document all data management activities and demonstrate compliance.
- Regularly update data management practices to align with the latest industry standards.
Data Security Concerns
Challenge:
Data management also involves handling sensitive patient information, making data security a top priority. Cybersecurity threats, unauthorized access, and data breaches can lead to serious legal and ethical issues.
Solutions:
- Encrypt all clinical trial data to protect it from unauthorized access.
- Implement role-based access controls (RBAC) to ensure only authorized personnel can view or modify specific data fields.
- Store clinical trial data in secure, cloud-based platforms with automated backups.
- Ensure compliance with HIPAA, GDPR, and other data privacy regulations to protect patient confidentiality.
Managing Large Volumes of Clinical Trial Data
Challenge:
The amount of data generated in clinical trials is increasing rapidly, making it difficult to manage, process, and analyze. Big data, wearable devices, real-time data streams, and electronic data capture (EDC) systems contribute to data overload.
Solutions:
- Use AI-driven analytics and machine learning to automate data cleaning and improve data consistency.
- Implement Oracle Clinical or similar advanced clinical data management systems for structured data handling.
- Standardize data collection formats to ensure data accuracy and easy integration across systems.
- Adopt secure cloud-based platforms for efficient data storage and quick retrieval of study data.
Overcoming these challenges with the right data management tools and strategies helps maintain high-quality data, regulatory compliance, and data integrity. Next, we’ll explore best practices for optimizing clinical data management practices to ensure that data remains accurate and secure.
Best Practices for Effective Clinical Data Management
Optimizing clinical data management practices is essential for ensuring data accuracy, integrity, and compliance throughout the clinical trial process. By implementing industry best practices, research teams can improve data quality, enhance efficiency, and reduce regulatory risks.
Below are key strategies that clinical research associates, data managers, and sponsors should follow.
1. Implement Robust EDC Systems for Accurate and Real-Time Data Collection
- Use Electronic Data Capture (EDC) systems to replace paper-based methods and minimize data entry errors.
- Enable real-time data capture from multiple sources, including wearable devices and remote patient monitoring tools.
- Ensure EDC systems comply with Clinical Data Interchange Standards Consortium (CDISC) guidelines for data standardization.
2. Conduct Regular Data Audits to Maintain Quality and Integrity
- Schedule periodic data quality checks to identify discrepancies and missing data.
- Implement an audit trail within the clinical data management system to track all data modifications.
- Follow Good Clinical Data Management Practices (GCDMP) to ensure that data remains complete and verifiable.
3. Utilize Automated Data Validation Tools to Minimize Errors
- Use built-in validation rules within EDC systems to detect outliers, missing values, and incorrect data entries.
- Apply double data entry verification for critical clinical trial data fields to enhance data accuracy.
- Implement machine learning algorithms for advanced error detection and data cleaning.
4. Train Research Teams on Data Management Protocols and Regulatory Requirements
- Provide ongoing training for data managers, clinical research associates (CRAs), and site staff on data management activities.
- Educate teams on CDISC, Clinical Data Acquisition Standards Harmonization (CDASH), HIPAA, and GDPR compliance requirements.
- Encourage participation in industry organizations such as the Society for Clinical Data Management (SCDM) to stay updated on best practices.
5. Ensure Compliance with Data Privacy Laws and Adopt Cybersecurity Measures
- Encrypt all clinical trial data to prevent unauthorized access.
- Implement role-based access controls (RBAC) to restrict access to sensitive study data.
- Use secure cloud-based clinical data management systems that comply with HIPAA, GDPR, and Good Clinical Practice (GCP) guidelines.
With these best practices, clinical research teams can maintain data integrity, improve data management efficiency, and ensure regulatory compliance. Next, let’s explore the future trends shaping clinical data management and how they will impact the industry.
Emerging Trends in Clinical Research Data Management
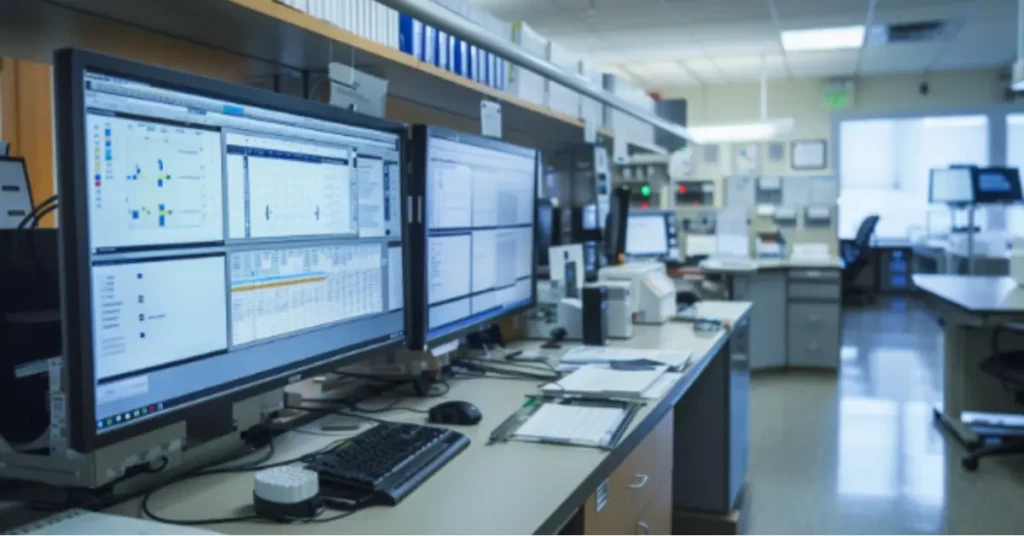
The field of clinical data management is rapidly evolving, driven by advancements in technology and the growing need for efficient data processing, security, and compliance. As clinical trials generate larger volumes of real-time data, the industry is shifting toward automation, decentralized models, and enhanced security measures to improve data accuracy, integrity, and efficiency.
Below are the key innovations shaping the future of data management in clinical research.
The Role of AI and Machine Learning in Data Management
Artificial intelligence (AI) and machine learning (ML) are transforming how clinical trial data is collected, validated, and analyzed. These technologies help ensure data accuracy, improve data consistency, and streamline data management activities.
- Automated Data Validation: AI-powered tools detect errors, outliers, and missing data in real-time, reducing manual workload.
- Predictive Analytics: Machine learning models identify patterns in study data, helping researchers anticipate potential issues before they impact the trial.
- Intelligent Data Cleaning: AI automates data cleaning processes by flagging inconsistencies and suggesting corrections, ensuring high-quality data for regulatory submissions.
By integrating AI-driven analytics into electronic data capture (EDC) systems and clinical data management systems (CDMS), research teams can process large volumes of clinical trial data faster and more accurately than ever before.
Blockchain for Data Integrity and Security
Ensuring data integrity is a major challenge in clinical trials, especially with concerns about data manipulation, unauthorized access, and regulatory compliance. Blockchain technology offers a solution by providing secure, tamper-proof, and transparent data management.
- Immutable Audit Trails: Blockchain records every transaction in a decentralized ledger, making it impossible to alter or delete study data without detection.
- Enhanced Data Security: Decentralized encryption ensures that sensitive clinical trial data remains protected from cyber threats.
- Smart Contracts for Compliance: Automated smart contracts enforce Good Clinical Practice (GCP) and regulatory standards, ensuring compliance with HIPAA, GDPR, and CDISC guidelines.
By adopting blockchain, the clinical research industry can enhance data security, transparency, and trust in the clinical trial process.
The Rise of Decentralized Clinical Trials (DCTs)
Decentralized clinical trials (DCTs) are changing how data collection and data management are conducted. Instead of requiring patients to visit central trial sites, DCTs use remote monitoring, wearable devices, and digital data collection systems to collect real-time participant data.
- Improved Data Capture: Digital health tools enable real-time data collection, reducing the risk of missing data and improving trial efficiency.
- Broader Patient Access: DCTs allow diverse patient populations to participate, increasing trial inclusivity and data diversity.
- Seamless Data Integration: Wearable devices and mobile health apps automatically sync with Clinical Data Management Systems (CDMS), ensuring efficient data processing and data consistency.
As more clinical trials adopt decentralized models, the clinical data management process must evolve to handle larger volumes of real-time data, maintain data integrity, and comply with regulatory requirements.
The future of clinical data management is being shaped by AI, blockchain, and decentralized trials, all of which aim to improve data quality, efficiency, and security. As these innovations continue to advance, research teams must adapt by implementing modern data management practices that align with these emerging trends.
The Importance of Robust Data Management in Clinical Research
Effective clinical data management is the backbone of successful clinical trials, ensuring data accuracy, regulatory compliance, and patient safety. From data collection and validation to storage and submission, every step must be meticulously managed to maintain data integrity and reliability.
Throughout this article, we’ve explored:
- The fundamentals of data management in clinical research, including its role in ensuring high-quality data.
- The step-by-step clinical data management process, from data capture to regulatory submission.
- Common challenges include data security risks, regulatory complexities, and managing large volumes of data, along with actionable solutions.
- Best practices to optimize clinical data management activities, such as using EDC systems, AI-driven validation, and regular data audits.
- Future trends are shaping the industry, including the rise of AI, blockchain, and decentralized clinical trials (DCTs).
As clinical trials become more data-intensive, investing in advanced data management solutions is no longer optional-it’s essential. Organizations prioritizing efficient data management practices will improve trial efficiency, accelerate regulatory approvals, and enhance patient safety.
To stay ahead, consider implementing modern clinical data management systems, adopting AI-driven analytics, and strengthening data security measures in your clinical research.